GEN AI in Action: How Product Owners Can Drive Innovation in Agile Teams"
One of the most significant challenges in integrating GEN AI is managing the complexity of the technology while maintaining a clear focus on product goals. In industries like healthcare and banking, where precision and compliance are paramount, Product Owners must navigate a delicate balance between innovation and regulation.
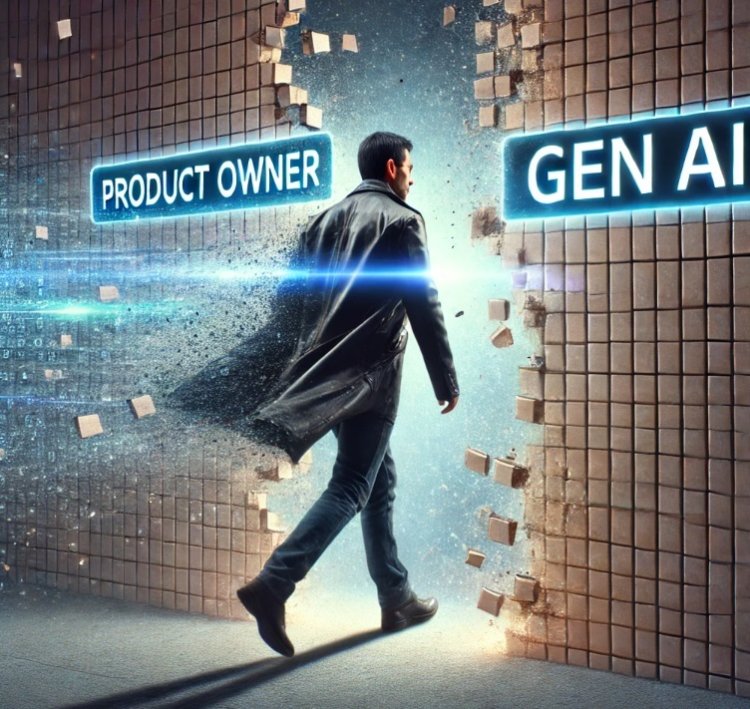
As a Product Owner, my journey has been deeply intertwined with both hardware and software. The emergence of Generative AI (GEN AI) is perhaps the most significant shift I've encountered in my career. Now, as we stand on the cusp of a new era, it’s crucial for Product Owners like us to grasp the tools and strategies that will define our roles in the age of GEN AI.
In this blog, I’ll dive into the impact of the best tools for Product Owners working with GEN AI. We’ll explore the necessary knowledge base, compare the leading tools, and see how they fit into agile methodologies, especially for Cloud-Based Microservices. As someone who’s equally passionate about robotics, software, and agile practices, I’ll share insights drawn from years of experience and provide a practical guide to navigating this evolving field.
Whether you’re working in manufacturing, healthcare, or any other industry, understanding GEN AI’s potential—and its challenges—is key to staying competitive. Let’s embark on this journey together, leveraging my experiences and the lessons learned along the way to equip you with the knowledge you need to excel in this rapidly changing environment.
GEN AI, or Generative AI, refers to a subset of artificial intelligence that focuses on creating new content, from text to images and even code. For Product Owners, understanding the basic terminology is essential. Terms like machine learning, deep learning, natural language processing, and transformers should be part of your everyday vocabulary. This foundation will not only help you communicate effectively with data scientists and developers but also enable you to make informed decisions about which tools and platforms are best suited for your projects.
Let’s begin by comparing some of the top GEN AI tools in the market. We’ll categorize them into three main groups: text generation, image generation, and code generation. Below is a comparative chart that highlights the strengths and weaknesses of each tool.
Tool | Category | Strengths | Weaknesses | Ideal Use Case |
---|---|---|---|---|
ChatGPT | Text Generation | Versatile, Natural Language Processing | Requires large datasets, Expensive | Customer service automation |
DALL-E | Image Generation | High-quality image synthesis | Computationally intensive | Marketing and content creation |
Codex | Code Generation | Supports multiple languages, Integrates with IDEs | Limited to specific languages | Software development assistance |
Below, The trend chart illustrates the rapid increase in GEN AI adoption across various industries, particularly healthcare and manufacturing. The data indicates that healthcare has seen the most significant growth due to the need for advanced diagnostic tools, while manufacturing is leveraging GEN AI for predictive maintenance and quality control.
Incorporating GEN AI into agile methodologies presents unique challenges and opportunities. Agile’s iterative nature aligns well with the flexibility of GEN AI, allowing for continuous improvement and rapid adaptation to market changes. However, Product Owners must ensure that the development team is fully onboarded with GEN AI principles and practices.
The flow diagram below illustrates how GEN AI can be integrated into an agile sprint cycle. From sprint planning to review, each stage incorporates feedback loops powered by GEN AI, ensuring that the product evolves in line with user needs and market demands. This flow diagram shows how GEN AI can enhance each phase of the agile process, from providing data-driven insights during sprint planning to automating testing and deployment tasks. It’s a powerful tool for maintaining agility while leveraging cutting-edge technology.
One of the most significant challenges in integrating GEN AI is managing the complexity of the technology while maintaining a clear focus on product goals. In industries like healthcare and banking, where precision and compliance are paramount, Product Owners must navigate a delicate balance between innovation and regulation.
The Pareto chart below identifies the top challenges faced by Product Owners when implementing GEN AI, with the most common issues being data privacy concerns, lack of skilled talent, and integration with existing systems. The Pareto chart reveals that a small number of challenges account for the majority of obstacles in GEN AI implementation. By focusing on these key areas—such as data privacy and talent acquisition—Product Owners can address the most critical issues first, paving the way for smoother integration.
Let’s look at how GEN AI is being utilized across various sectors:
-
Manufacturing: Predictive maintenance, powered by GEN AI, is revolutionizing the way companies like Bimbo operate their automated production lines.
-
Healthcare: In hospitals, GEN AI is enhancing patient care through personalized treatment plans and early diagnosis of diseases.
-
Transportation and Logistics: Autonomous vehicles and route optimization are just the beginning of what GEN AI can do in this field.
-
Banking: Fraud detection and risk assessment are areas where GEN AI is making significant inroads, ensuring safer transactions.
-
Retail: Personalized shopping experiences driven by GEN AI are reshaping the consumer landscape, from product recommendations to supply chain management.
GEN AI tools can seamlessly fit into agile methodologies, particularly in cloud-based microservices environments. For instance, using AI-driven tools for automated testing can drastically reduce the time spent on quality assurance, allowing for faster iterations and more efficient sprints. Moreover, AI’s predictive capabilities can help in backlog prioritization, ensuring that the most critical features are developed first.
Looking to the future, the next five years will likely see GEN AI becoming even more integrated into the fabric of product management. From onboarding teams to optimizing SaaS platforms, the potential is vast. However, success will depend on the Product Owner’s ability to stay informed and adaptable.
To stay ahead, obtaining certifications in GEN AI and related fields is crucial. Certifications such as Google’s AI Certification, AWS Certified Machine Learning, and Stanford’s AI Professional Program can provide a solid foundation. Moreover, skills in data science, agile methodologies, and cloud platforms will be invaluable.
Drawing from my journey through engineering and product management, I've seen how innovation can transform industries. GEN AI is no exception. Whether you're in healthcare, manufacturing, retail, or any other sector, the future belongs to those who can navigate the complexities of this technology and lead their teams with confidence.
Through my experiences, I’ve learned that the key to success lies in a deep understanding of both technology and people. I hope the basics provided in this blog help you motivate to learn more about the complexities of GEN AI and lead your teams to new heights of achievement.
Thank you for reading! If you found this blog insightful, I’d love for you to share it with your network on social media. Don’t forget to leave your thoughts, comments, and suggestions below—I’m eager to hear from you. If you enjoyed this article, please click ‘like’ and consider subscribing for more content like this. For further discussion or inquiries, feel free to reach out to me via email. Let’s continue the conversation and explore how GEN AI can shape the future of product management together.
Gartner, Inc. (2023). "Top Trends in Artificial Intelligence." McKinsey & Company. (2023). "The State of AI in 2023: Accelerating Advancements." Product Management Institute. (2023). "Challenges of Implementing GEN AI in Agile Methodologies." Forrester Research. (2023). "The Impact of GEN AI on Product Development." Google AI. (2024). "Introduction to AI and Machine Learning." Amazon Web Services. (2024). "Machine Learning on AWS." Stanford University. (2024). "AI Professional Program." MIT. (2023). "Research on AI Adoption and Operational Efficiency." Agile Alliance. (2024). "Integrating AI into Agile Methodologies." Industry White Papers. (2024). "Future Trends in GEN AI."
What's Your Reaction?
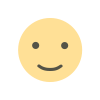
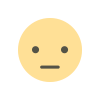

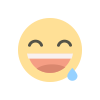
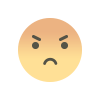
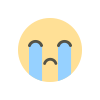
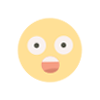